Don't Waste Your Data: Using Tracking Data to Find Key Moments in Soccer with Open Space
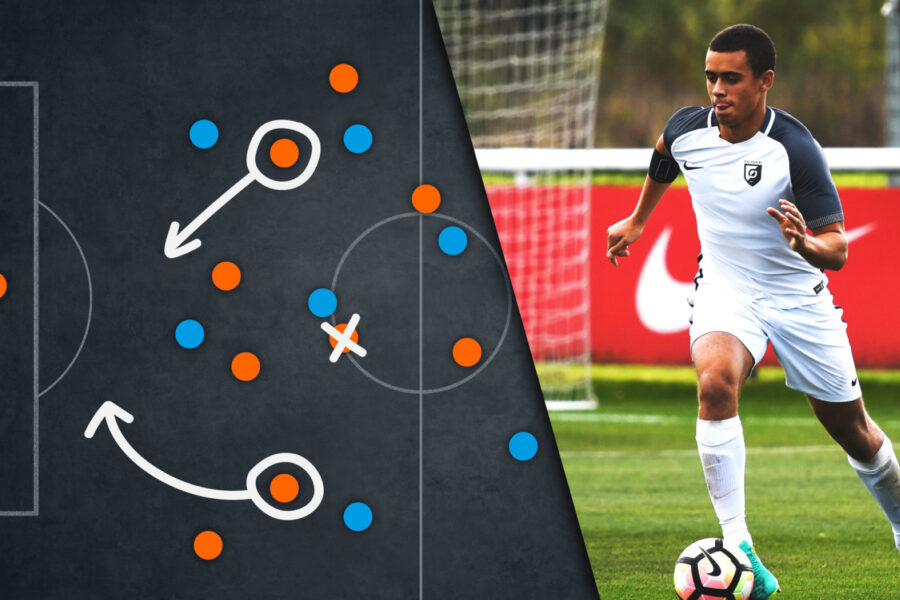
Our research and development team was selected to present at the 2017 OptaPro & MIT Sloan Analytics Conferences on their recent work analyzing passing probabilities in football (soccer).
At Hudl, we believe more than anyone that understanding matches is always tied back to video. Accordingly, when we build analytical models, our intent is to help our analyst colleagues identify and highlight moments in video that can be used to prepare for the next match or learn from a previous one.
Moment Discovery
This year at the MIT Sloan Sports Analytics Conference, I was honored to present a research paper about passing probabilities in football. The passing model allows us to compute the probability that a pass will succeed or fail and which player is best situated to receive the pass. This answers many questions when tied back to video.
For example, if we’re interested in knowing how the opponent was able to create dangerous attacks, we can look for situations in which a high probability pass was completed to their striker and/or winger in the attacking third of the pitch. By highlighting these moments, we can analyze the video to determine how such a high probability pass was allowed to occur in the first place.
Adding Context
In addition to finding moments in the video, we can add context to sequences of events. This could be used to augment our ability to find interesting moments, or to highlight information about a sequence of events. Let’s take a look at a specific example to illustrate how knowing the probability of a pass can add important context to a sequence of events.
This data comes from a professional men’s league. We have anonymized the the data and will refer to players from the Red Team and the Blue Team by their randomized “jersey” numbers. The Blue Team is attacking right to left and has possession of the ball. Its first pass is from defender Blue #26 who directs a pass to midfielder Blue #17.
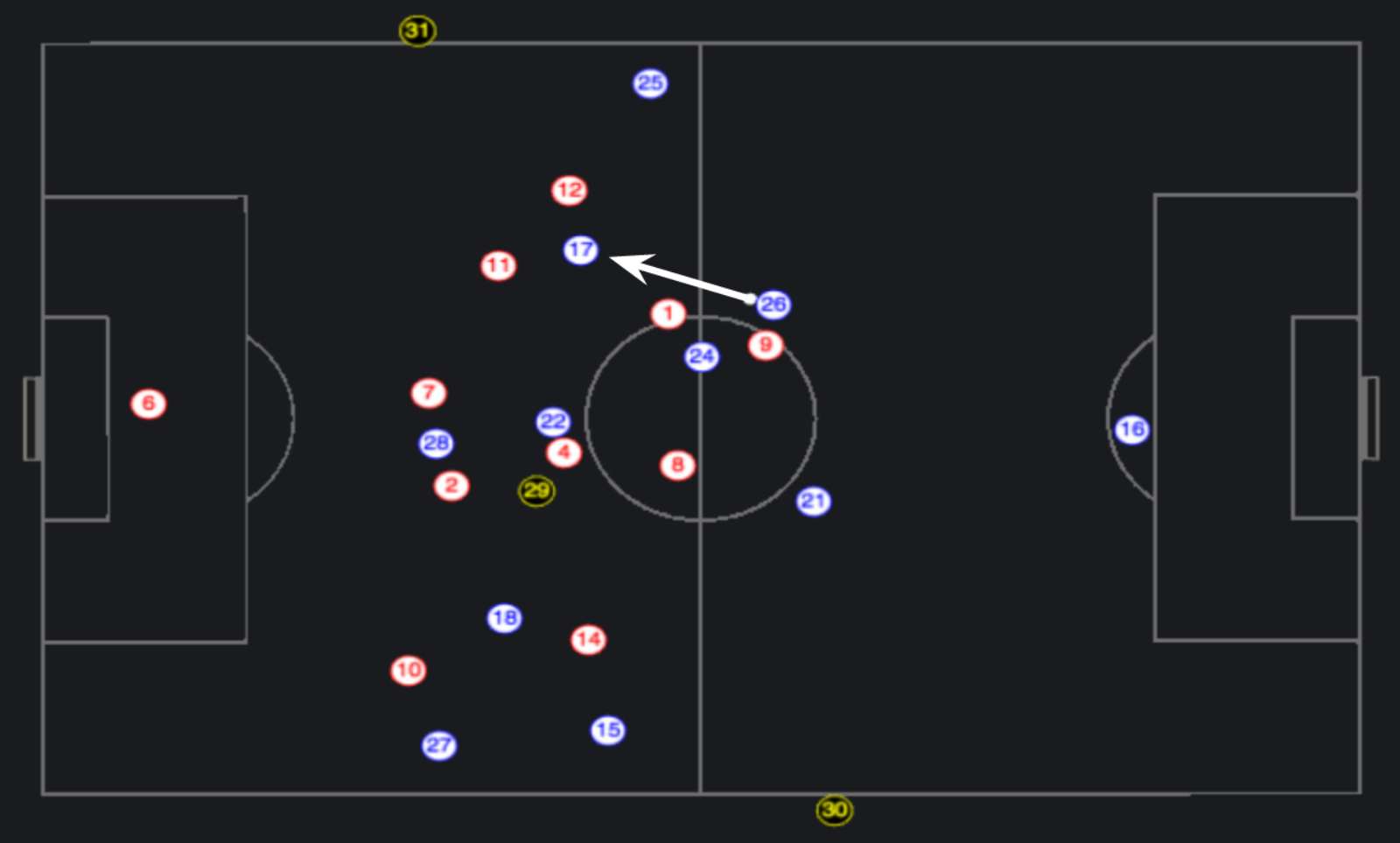
This is a fairly easy pass but the presence of Red #1, who could intercept, and Red #11 and Red #12, who could challenge, mean that the receiver must quickly control the ball if he’s going to keep possession. Blue #17 runs back and chips a short pass to Blue #24. This is an easy pass and has an 85% chance of success.
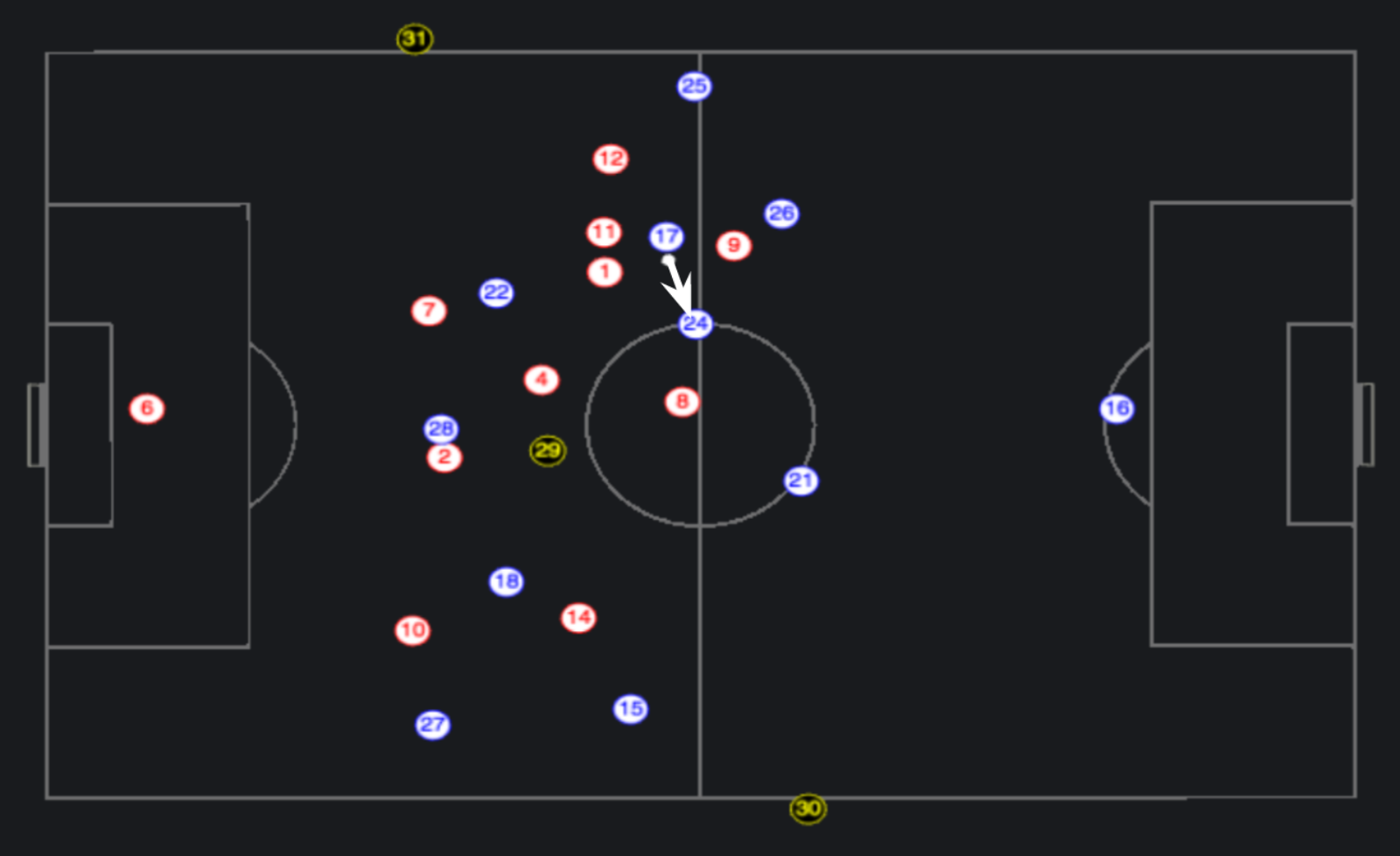
As Red #8 closes, Blue #24 pivots and notices that Blue #28 is in excellent scoring position. Blue #24 sends a precise ball deep to Blue #28. This is a highly valuable pass because it could result in a strong scoring chance, even though it is unlikely to be completed due to the presence of defenders (Red #2 and Red #7) and the goalkeeper.
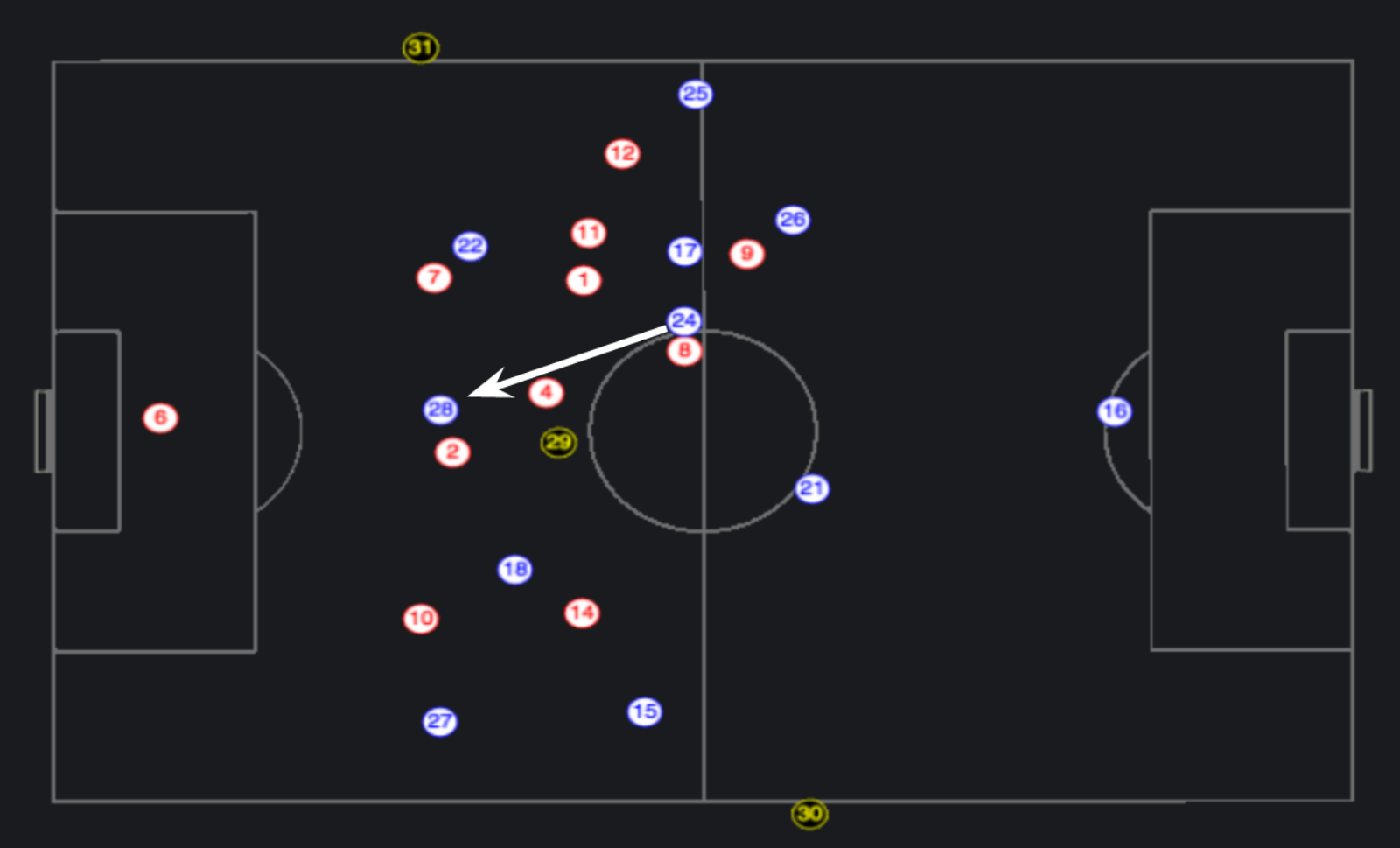
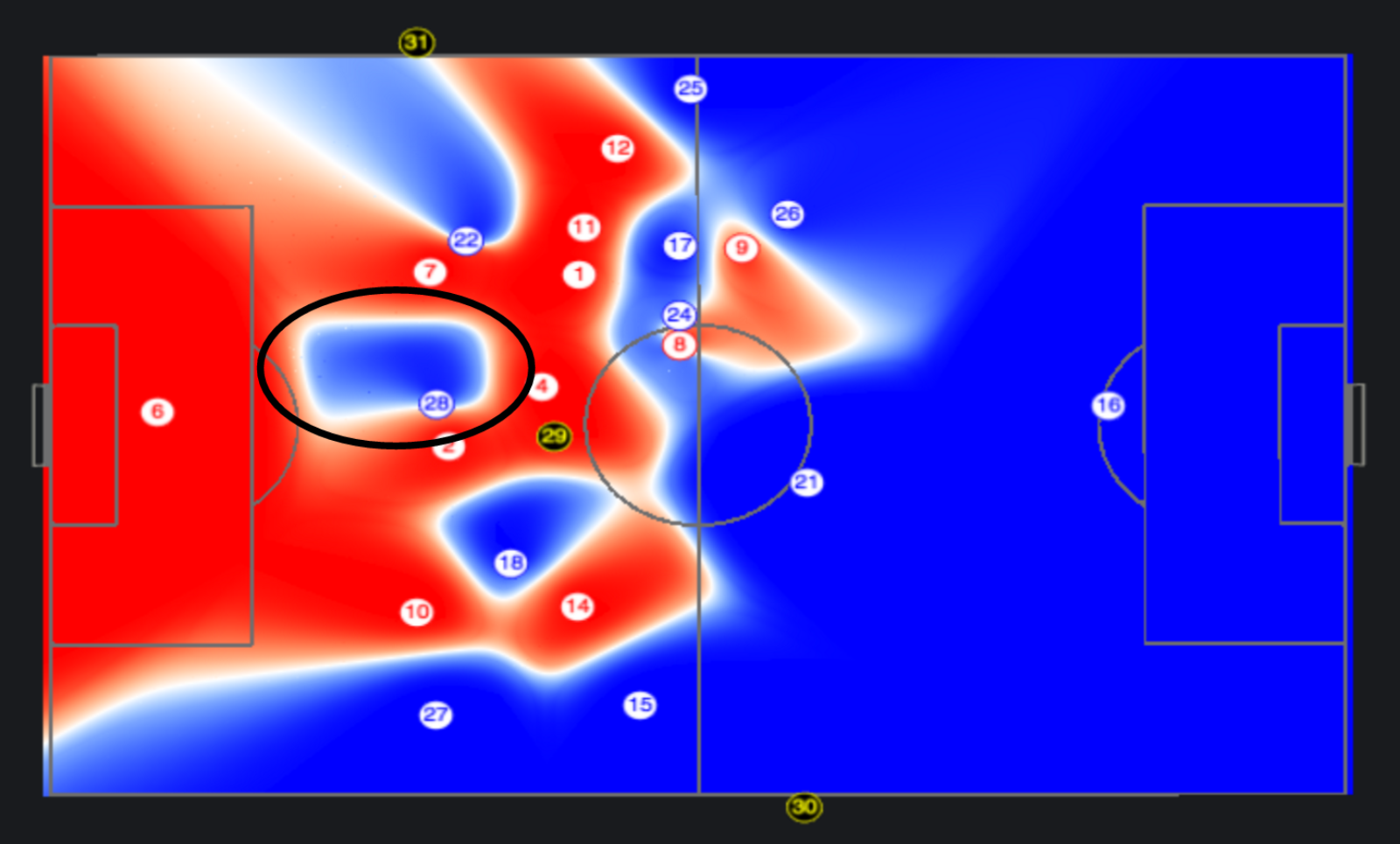
Open Space Analysis
Using this open space model, we can visualize how the pass to Blue #28 became possible in the first place. Looking at the open space model, we can see that Blue #22 is moving down the right side of the pitch. This draws away defender Red #7, which opens up space to the right of striker Blue #28. Defender Red #2 could be positioned closer to the goal, which would cut off Blue #28 from having an open lane, but such positioning would give Blue #18 and Blue #27 a 2-on-1 matchup on the left side of the pitch.
The last pass in the pass-chain leading up to the score had a mere 26% chance of success, but even if a goal had not been scored, this would be a sequence of events that could be analyzed after the match. Because we can compute the pass probabilities, we can identify similar dangerous opportunities to pass into the box that represent a scoring chance even if no actual pass or shot takes place.
Conclusions
By pairing video with tracking data, we can assist analysts in finding and evaluating critical moments during a match.
These models not only add a new dimension of analysis to the data, but also make it faster and easier than ever to find key moments in video for analysts to review. Teams are no longer limited to on-ball events, as we can use these models to find opportunities even if they were not exploited during the match.
We’re excited to announce our research in passing and spatial analysis, and we look forward to using these tools to streamline how you can engage with your data. If you would like to incorporate these tools into your workflow, please let us know.
If you’re interested in learning more about our research efforts in football, follow me on Twitter or reach out to our product manager, Armen Badeer.